注記
完全なサンプルコードをダウンロードするには、最後まで移動してください。または、Binder経由でブラウザでこの例を実行するには
セグメンテーション指標の評価#
異なるセグメンテーション手法を試す際に、どれが最適かを知るにはどうすればよいでしょうか? *グランドトゥルース* または *ゴールドスタンダード* セグメンテーションがある場合、さまざまな指標を使用して、各自動化手法がどの程度真実に近いかを確認できます。この例では、セグメント化しやすい画像を使用して、さまざまなセグメンテーション指標の解釈方法を示します。例として、適合Rand誤差と情報の変動を使用し、*過剰セグメンテーション* (真のセグメントを過剰なサブセグメントに分割すること)と *過少セグメンテーション* (異なる真のセグメントを単一のセグメントにマージすること)が異なるスコアにどのように影響するかを確認します。
import numpy as np
import matplotlib.pyplot as plt
from scipy import ndimage as ndi
from skimage import data
from skimage.metrics import adapted_rand_error, variation_of_information
from skimage.filters import sobel
from skimage.measure import label
from skimage.util import img_as_float
from skimage.feature import canny
from skimage.morphology import remove_small_objects
from skimage.segmentation import (
morphological_geodesic_active_contour,
inverse_gaussian_gradient,
watershed,
mark_boundaries,
)
image = data.coins()
まず、真のセグメンテーションを生成します。この単純な画像の場合、完全なセグメンテーションを生成する正確な関数とパラメータがわかっています。実際のシナリオでは、通常、手動アノテーションまたはセグメンテーションの「ペイント」によってグランドトゥルースを生成します。
elevation_map = sobel(image)
markers = np.zeros_like(image)
markers[image < 30] = 1
markers[image > 150] = 2
im_true = watershed(elevation_map, markers)
im_true = ndi.label(ndi.binary_fill_holes(im_true - 1))[0]
次に、異なる特性を持つ3つの異なるセグメンテーションを作成します。最初のものは、*コンパクトネス* を使用した skimage.segmentation.watershed()
を使用します。これは有用な初期セグメンテーションですが、最終結果としては細かすぎます。これが過剰セグメンテーション指標を急上昇させる様子を見ていきます。
次のアプローチでは、Cannyエッジフィルター skimage.feature.canny()
を使用します。これは非常に優れたエッジファインダーであり、バランスの取れた結果をもたらします。
edges = canny(image)
fill_coins = ndi.binary_fill_holes(edges)
im_test2 = ndi.label(remove_small_objects(fill_coins, 21))[0]
最後に、モルフォロジカル測地線アクティブコンター skimage.segmentation.morphological_geodesic_active_contour()
を使用します。これは一般的に良好な結果をもたらしますが、適切な解に収束するには長い時間がかかります。意図的に手順を100反復で打ち切っているので、最終結果は *過少セグメント化* されます。つまり、多くの領域が1つのセグメントにマージされます。セグメンテーション指標に対する対応する影響を見ていきます。
image = img_as_float(image)
gradient = inverse_gaussian_gradient(image)
init_ls = np.zeros(image.shape, dtype=np.int8)
init_ls[10:-10, 10:-10] = 1
im_test3 = morphological_geodesic_active_contour(
gradient,
num_iter=100,
init_level_set=init_ls,
smoothing=1,
balloon=-1,
threshold=0.69,
)
im_test3 = label(im_test3)
method_names = [
'Compact watershed',
'Canny filter',
'Morphological Geodesic Active Contours',
]
short_method_names = ['Compact WS', 'Canny', 'GAC']
precision_list = []
recall_list = []
split_list = []
merge_list = []
for name, im_test in zip(method_names, [im_test1, im_test2, im_test3]):
error, precision, recall = adapted_rand_error(im_true, im_test)
splits, merges = variation_of_information(im_true, im_test)
split_list.append(splits)
merge_list.append(merges)
precision_list.append(precision)
recall_list.append(recall)
print(f'\n## Method: {name}')
print(f'Adapted Rand error: {error}')
print(f'Adapted Rand precision: {precision}')
print(f'Adapted Rand recall: {recall}')
print(f'False Splits: {splits}')
print(f'False Merges: {merges}')
fig, axes = plt.subplots(2, 3, figsize=(9, 6), constrained_layout=True)
ax = axes.ravel()
ax[0].scatter(merge_list, split_list)
for i, txt in enumerate(short_method_names):
ax[0].annotate(txt, (merge_list[i], split_list[i]), verticalalignment='center')
ax[0].set_xlabel('False Merges (bits)')
ax[0].set_ylabel('False Splits (bits)')
ax[0].set_title('Split Variation of Information')
ax[1].scatter(precision_list, recall_list)
for i, txt in enumerate(short_method_names):
ax[1].annotate(txt, (precision_list[i], recall_list[i]), verticalalignment='center')
ax[1].set_xlabel('Precision')
ax[1].set_ylabel('Recall')
ax[1].set_title('Adapted Rand precision vs. recall')
ax[1].set_xlim(0, 1)
ax[1].set_ylim(0, 1)
ax[2].imshow(mark_boundaries(image, im_true))
ax[2].set_title('True Segmentation')
ax[2].set_axis_off()
ax[3].imshow(mark_boundaries(image, im_test1))
ax[3].set_title('Compact Watershed')
ax[3].set_axis_off()
ax[4].imshow(mark_boundaries(image, im_test2))
ax[4].set_title('Edge Detection')
ax[4].set_axis_off()
ax[5].imshow(mark_boundaries(image, im_test3))
ax[5].set_title('Morphological GAC')
ax[5].set_axis_off()
plt.show()
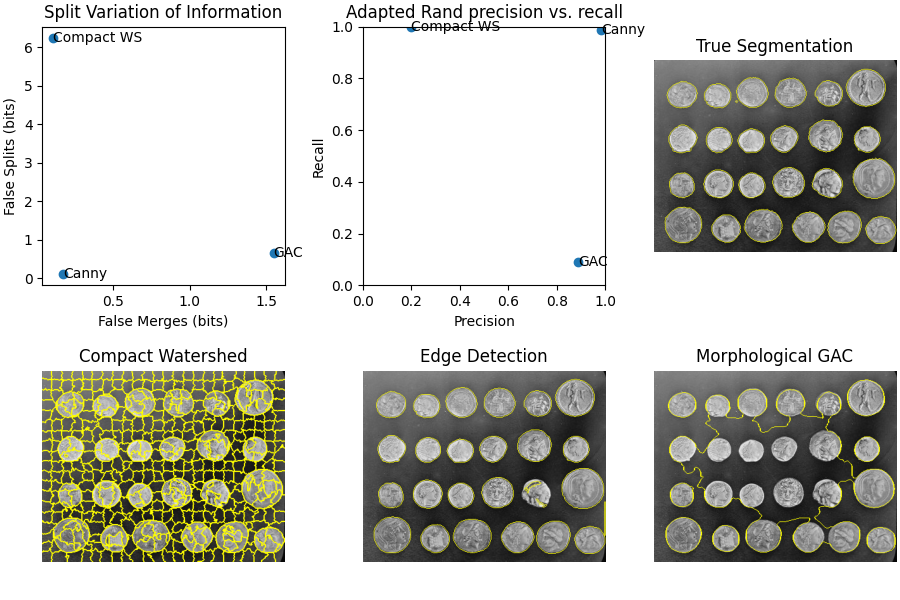
## Method: Compact watershed
Adapted Rand error: 0.6696040824674964
Adapted Rand precision: 0.19789866776616444
Adapted Rand recall: 0.999747618854942
False Splits: 6.235700763571577
False Merges: 0.10855347108404328
## Method: Canny filter
Adapted Rand error: 0.01477976422795424
Adapted Rand precision: 0.9829789913922441
Adapted Rand recall: 0.9874717238207291
False Splits: 0.11790842956314956
False Merges: 0.17056628682718059
## Method: Morphological Geodesic Active Contours
Adapted Rand error: 0.8380351755097508
Adapted Rand precision: 0.8878701134692834
Adapted Rand recall: 0.08911012454398565
False Splits: 0.6563768831046463
False Merges: 1.5482985465714199
**スクリプトの合計実行時間:** (0分3.510秒)