ノート
フルサンプルコードをダウンロードするまたはBinder経由でブラウザでこのサンプルを実行するには、最後まで進んでください。
画像のノイズ除去#
この例では、全変動、バイラテラル、およびウェーブレットノイズ除去フィルターを使用して、ノイズの多い画像のノイズを除去します。
全変動アルゴリズムとバイラテラルアルゴリズムは、通常、鋭いエッジで区切られた平坦な領域を持つ「ポスタライズ」された画像を生成します。ノイズ除去と元の画像への忠実さの間のトレードオフを制御することで、ポスタライズの程度を変更できます。
全変動フィルター#
このフィルターの結果は、可能な限り元の画像に近い状態を保ちながら、最小限の全変動ノルムを持つ画像です。全変動は、画像の勾配のL1ノルムです。
バイラテラルフィルター#
バイラテラルフィルターは、エッジを保持しノイズを低減するフィルターです。空間的な近さと放射線的な類似性に基づいてピクセルを平均化します。
ウェーブレットノイズ除去フィルター#
ウェーブレットノイズ除去フィルターは、画像のウェーブレット表現に依存します。ノイズは、ウェーブレットドメインの小さな値で表され、0に設定されます。
カラー画像では、ウェーブレットノイズ除去は通常、YCbCr色空間で行われます。これは、個別のカラーチャネルでノイズ除去を行うと、ノイズがより顕著になる可能性があるためです。
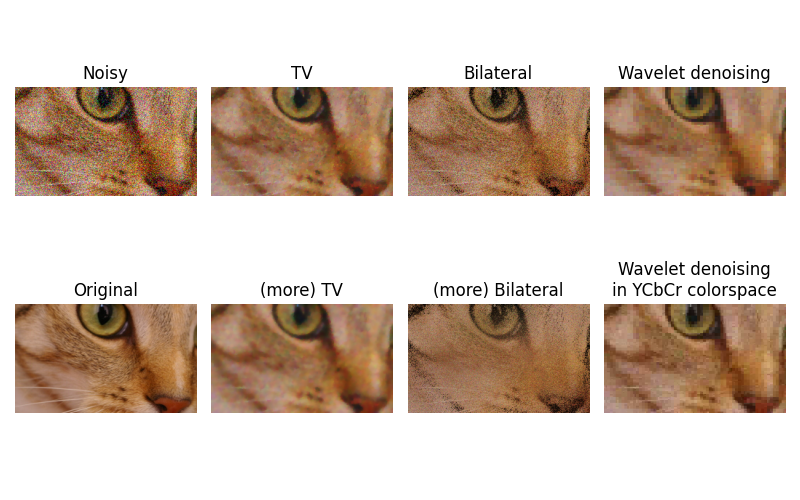
Estimated Gaussian noise standard deviation = 0.1496192774329601
Clipping input data to the valid range for imshow with RGB data ([0..1] for floats or [0..255] for integers). Got range [-0.002508543763914225..0.8470964845245508].
import matplotlib.pyplot as plt
from skimage.restoration import (
denoise_tv_chambolle,
denoise_bilateral,
denoise_wavelet,
estimate_sigma,
)
from skimage import data, img_as_float
from skimage.util import random_noise
original = img_as_float(data.chelsea()[100:250, 50:300])
sigma = 0.155
noisy = random_noise(original, var=sigma**2)
fig, ax = plt.subplots(nrows=2, ncols=4, figsize=(8, 5), sharex=True, sharey=True)
plt.gray()
# Estimate the average noise standard deviation across color channels.
sigma_est = estimate_sigma(noisy, channel_axis=-1, average_sigmas=True)
# Due to clipping in random_noise, the estimate will be a bit smaller than the
# specified sigma.
print(f'Estimated Gaussian noise standard deviation = {sigma_est}')
ax[0, 0].imshow(noisy)
ax[0, 0].axis('off')
ax[0, 0].set_title('Noisy')
ax[0, 1].imshow(denoise_tv_chambolle(noisy, weight=0.1, channel_axis=-1))
ax[0, 1].axis('off')
ax[0, 1].set_title('TV')
ax[0, 2].imshow(
denoise_bilateral(noisy, sigma_color=0.05, sigma_spatial=15, channel_axis=-1)
)
ax[0, 2].axis('off')
ax[0, 2].set_title('Bilateral')
ax[0, 3].imshow(denoise_wavelet(noisy, channel_axis=-1, rescale_sigma=True))
ax[0, 3].axis('off')
ax[0, 3].set_title('Wavelet denoising')
ax[1, 1].imshow(denoise_tv_chambolle(noisy, weight=0.2, channel_axis=-1))
ax[1, 1].axis('off')
ax[1, 1].set_title('(more) TV')
ax[1, 2].imshow(
denoise_bilateral(noisy, sigma_color=0.1, sigma_spatial=15, channel_axis=-1)
)
ax[1, 2].axis('off')
ax[1, 2].set_title('(more) Bilateral')
ax[1, 3].imshow(
denoise_wavelet(noisy, channel_axis=-1, convert2ycbcr=True, rescale_sigma=True)
)
ax[1, 3].axis('off')
ax[1, 3].set_title('Wavelet denoising\nin YCbCr colorspace')
ax[1, 0].imshow(original)
ax[1, 0].axis('off')
ax[1, 0].set_title('Original')
fig.tight_layout()
plt.show()
スクリプトの合計実行時間: (0分12.432秒)