注記
最後まで進むと、完全なサンプルコードをダウンロードできます。または、Binder経由でブラウザでこの例を実行するには、
ウォーターシェッド分割#
ウォーターシェッドは、**セグメンテーション**、つまり画像内の異なるオブジェクトを分離するために使用される古典的なアルゴリズムです。
ユーザー定義のマーカーから開始して、ウォーターシェッドアルゴリズムはピクセル値を局所的な地形(標高)として扱います。アルゴリズムは、異なるマーカーに起因する流域が分水嶺線上で出会うまで、マーカーから流域を氾濫させます。多くの場合、マーカーは画像の局所的最小値として選択され、そこから流域が氾濫します。
以下の例では、2つの重なり合う円を分離します。そのためには、背景までの距離である画像を計算します。この距離の最大値(つまり、距離の反対の最小値)がマーカーとして選択され、そのようなマーカーからの流域の氾濫は分水嶺線に沿って2つの円を分離します。
アルゴリズムの詳細については、Wikipediaを参照してください。
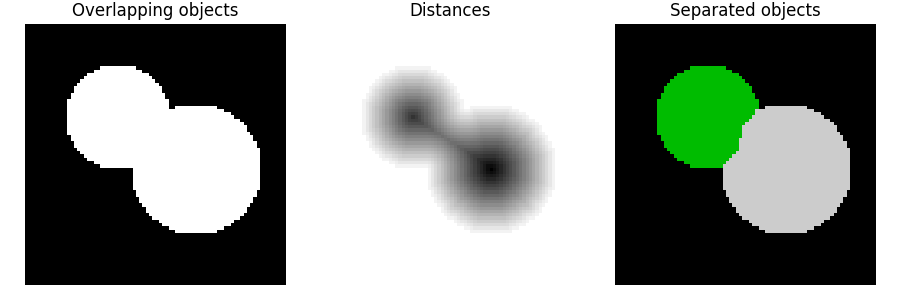
import numpy as np
import matplotlib.pyplot as plt
from scipy import ndimage as ndi
from skimage.segmentation import watershed
from skimage.feature import peak_local_max
# Generate an initial image with two overlapping circles
x, y = np.indices((80, 80))
x1, y1, x2, y2 = 28, 28, 44, 52
r1, r2 = 16, 20
mask_circle1 = (x - x1) ** 2 + (y - y1) ** 2 < r1**2
mask_circle2 = (x - x2) ** 2 + (y - y2) ** 2 < r2**2
image = np.logical_or(mask_circle1, mask_circle2)
# Now we want to separate the two objects in image
# Generate the markers as local maxima of the distance to the background
distance = ndi.distance_transform_edt(image)
coords = peak_local_max(distance, footprint=np.ones((3, 3)), labels=image)
mask = np.zeros(distance.shape, dtype=bool)
mask[tuple(coords.T)] = True
markers, _ = ndi.label(mask)
labels = watershed(-distance, markers, mask=image)
fig, axes = plt.subplots(ncols=3, figsize=(9, 3), sharex=True, sharey=True)
ax = axes.ravel()
ax[0].imshow(image, cmap=plt.cm.gray)
ax[0].set_title('Overlapping objects')
ax[1].imshow(-distance, cmap=plt.cm.gray)
ax[1].set_title('Distances')
ax[2].imshow(labels, cmap=plt.cm.nipy_spectral)
ax[2].set_title('Separated objects')
for a in ax:
a.set_axis_off()
fig.tight_layout()
plt.show()
**スクリプトの総実行時間:**(0分0.169秒)