注記
最後まで移動して、完全なサンプルコードをダウンロードします。または、Binder経由でブラウザでこの例を実行します。
平均フィルター#
この例では、ランクフィルターパッケージの以下の平均フィルターを比較します。
局所平均:平均グレースケールを計算するための構造要素に属するすべてのピクセル。
パーセンタイル平均:パーセンタイルp0とp1(ここでは10%と90%)の間の値のみを使用します。
双方向平均:g-s0とg+s1(ここではg-500とg+500)の範囲内にあるグレースケールを持つ構造要素のピクセルのみを使用します。
パーセンタイル平均と通常の平均はここで同様の結果を与え、これらのフィルターは画像全体(背景と詳細)を平滑化します。双方向平均は連続領域(つまり背景)に対して高いフィルタリングレートを示す一方で、より高い画像周波数はそのまま残ります。
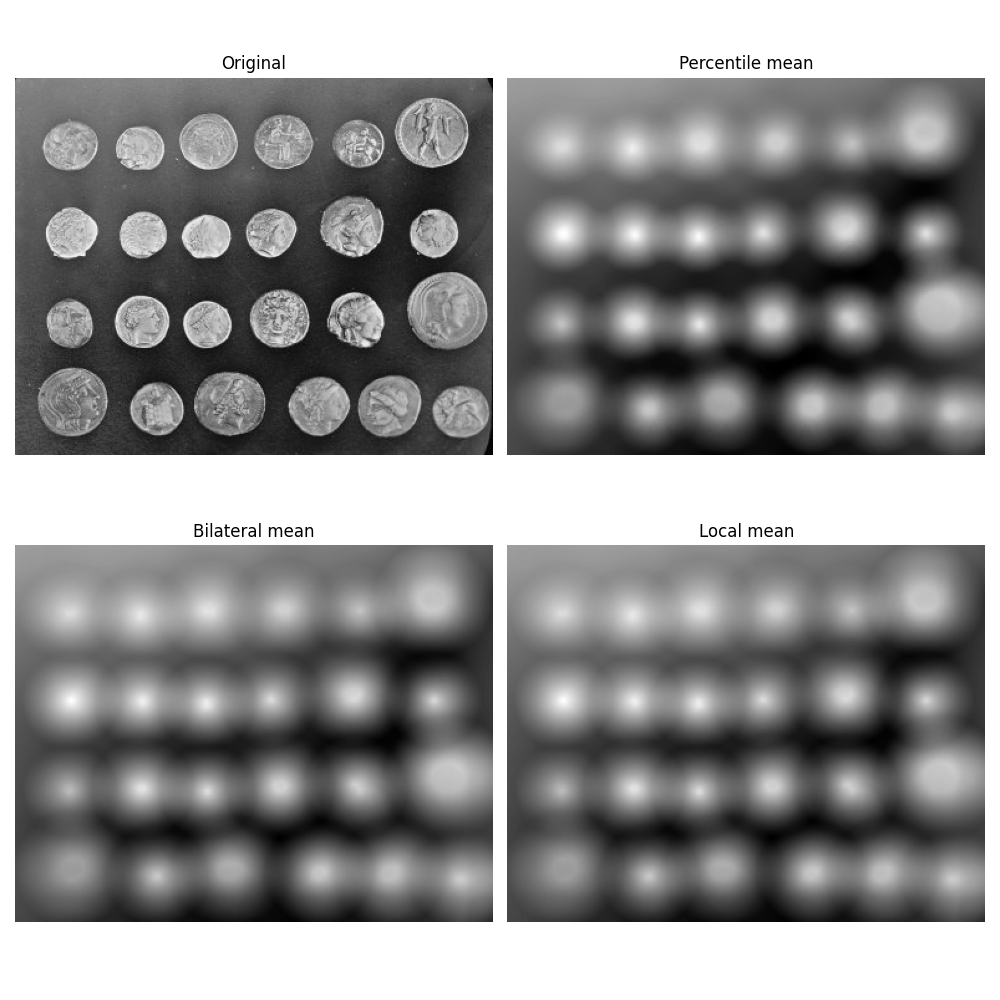
import matplotlib.pyplot as plt
from skimage import data
from skimage.morphology import disk
from skimage.filters import rank
image = data.coins()
footprint = disk(20)
percentile_result = rank.mean_percentile(image, footprint=footprint, p0=0.1, p1=0.9)
bilateral_result = rank.mean_bilateral(image, footprint=footprint, s0=500, s1=500)
normal_result = rank.mean(image, footprint=footprint)
fig, axes = plt.subplots(nrows=2, ncols=2, figsize=(10, 10), sharex=True, sharey=True)
ax = axes.ravel()
titles = ['Original', 'Percentile mean', 'Bilateral mean', 'Local mean']
imgs = [image, percentile_result, bilateral_result, normal_result]
for n in range(0, len(imgs)):
ax[n].imshow(imgs[n], cmap=plt.cm.gray)
ax[n].set_title(titles[n])
ax[n].axis('off')
plt.tight_layout()
plt.show()
スクリプトの総実行時間:(0分1.547秒)