注記
最後まで移動して、完全な例コードをダウンロードしてください。または、Binder経由でブラウザでこの例を実行してください。
テクスチャを保持する非局所平均ノイズ除去#
この例では、非局所平均フィルタを使用して、宇宙飛行士画像の詳細部分をノイズ除去します。非局所平均アルゴリズムは、ピクセルの値を他のピクセルの値の平均で置き換えます。他のピクセルを中心とした小さなパッチが、対象のピクセルを中心としたパッチと比較され、平均は現在のパッチに近いパッチを持つピクセルに対してのみ実行されます。その結果、このアルゴリズムは、他のノイズ除去アルゴリズムではぼやけてしまうテクスチャをうまく復元できます。
fast_mode
引数がFalse
の場合、パッチ間の距離を計算する際に、空間ガウシアン重みがパッチに適用されます。fast_mode
がTrue
の場合、パッチに対して一様な空間重み付けを使用するより高速なアルゴリズムが適用されます。
いずれの場合も、ノイズの標準偏差sigma
が指定されている場合、パッチ間の距離を計算する際に、期待されるノイズ分散が差し引かれます。これにより、画像品質がわずかに向上する可能性があります。
estimate_sigma
関数は、非局所平均アルゴリズムのパラメータh
(およびオプションでsigma
)を設定するための良い出発点となります。h
は、パッチ間の距離の関数としてパッチの重みの減衰を制御する定数です。より大きなh
は、類似性の低いパッチ間の平滑化をより多く許可します。
このデモでは、h
は、各バリアントのおおよその最良性能を得るように手動で調整されました。
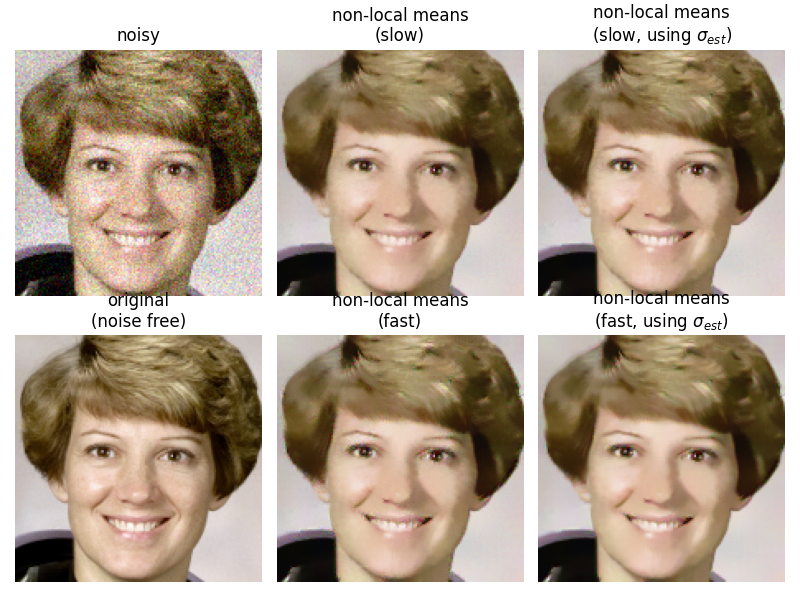
estimated noise standard deviation = 0.07824735983398969
PSNR (noisy) = 22.21
PSNR (slow) = 29.30
PSNR (slow, using sigma) = 29.72
PSNR (fast) = 28.88
PSNR (fast, using sigma) = 29.26
import numpy as np
import matplotlib.pyplot as plt
from skimage import data, img_as_float
from skimage.restoration import denoise_nl_means, estimate_sigma
from skimage.metrics import peak_signal_noise_ratio
from skimage.util import random_noise
astro = img_as_float(data.astronaut())
astro = astro[30:180, 150:300]
sigma = 0.08
noisy = random_noise(astro, var=sigma**2)
# estimate the noise standard deviation from the noisy image
sigma_est = np.mean(estimate_sigma(noisy, channel_axis=-1))
print(f'estimated noise standard deviation = {sigma_est}')
patch_kw = dict(
patch_size=5, # 5x5 patches
patch_distance=6, # 13x13 search area
channel_axis=-1,
)
# slow algorithm
denoise = denoise_nl_means(noisy, h=1.15 * sigma_est, fast_mode=False, **patch_kw)
# slow algorithm, sigma provided
denoise2 = denoise_nl_means(
noisy, h=0.8 * sigma_est, sigma=sigma_est, fast_mode=False, **patch_kw
)
# fast algorithm
denoise_fast = denoise_nl_means(noisy, h=0.8 * sigma_est, fast_mode=True, **patch_kw)
# fast algorithm, sigma provided
denoise2_fast = denoise_nl_means(
noisy, h=0.6 * sigma_est, sigma=sigma_est, fast_mode=True, **patch_kw
)
fig, ax = plt.subplots(nrows=2, ncols=3, figsize=(8, 6), sharex=True, sharey=True)
ax[0, 0].imshow(noisy)
ax[0, 0].axis('off')
ax[0, 0].set_title('noisy')
ax[0, 1].imshow(denoise)
ax[0, 1].axis('off')
ax[0, 1].set_title('non-local means\n(slow)')
ax[0, 2].imshow(denoise2)
ax[0, 2].axis('off')
ax[0, 2].set_title('non-local means\n(slow, using $\\sigma_{est}$)')
ax[1, 0].imshow(astro)
ax[1, 0].axis('off')
ax[1, 0].set_title('original\n(noise free)')
ax[1, 1].imshow(denoise_fast)
ax[1, 1].axis('off')
ax[1, 1].set_title('non-local means\n(fast)')
ax[1, 2].imshow(denoise2_fast)
ax[1, 2].axis('off')
ax[1, 2].set_title('non-local means\n(fast, using $\\sigma_{est}$)')
fig.tight_layout()
# print PSNR metric for each case
psnr_noisy = peak_signal_noise_ratio(astro, noisy)
psnr = peak_signal_noise_ratio(astro, denoise)
psnr2 = peak_signal_noise_ratio(astro, denoise2)
psnr_fast = peak_signal_noise_ratio(astro, denoise_fast)
psnr2_fast = peak_signal_noise_ratio(astro, denoise2_fast)
print(f'PSNR (noisy) = {psnr_noisy:0.2f}')
print(f'PSNR (slow) = {psnr:0.2f}')
print(f'PSNR (slow, using sigma) = {psnr2:0.2f}')
print(f'PSNR (fast) = {psnr_fast:0.2f}')
print(f'PSNR (fast, using sigma) = {psnr2_fast:0.2f}')
plt.show()
**スクリプトの実行時間合計:**(0分2.921秒)