注記
完全なコード例をダウンロードするには、最後まで進んでください。または、Binder経由でブラウザでこの例を実行するには、
画像ワーピングのための薄板スプラインの使用#
画像をワーピングするには、ソース座標とターゲット座標のセットから始めます。目標は、ソース点がターゲット位置に移動するように画像を変形することです。通常、少数の選択されたソース点のターゲット位置のみがわかっています。他のすべてのピクセル位置のターゲット位置を計算するには、モデルが必要です。アフィン変換や射影変換など、さまざまなモデルが存在します。
ほとんどの変換は線形ですが(つまり、直線を保持します)、より柔軟性が必要な場合があります。線形ではない変換、つまり線が曲がる可能性のある変換を表す1つのモデルは、薄板スプラインです[1] [2]。
薄板スプラインは、固有の剛性を持つ金属板のアナロジーに基づいています。ソース点について考えてみましょう。各ソース点は、対応するターゲット位置に着地するために、x方向とy方向の両方で一定の距離を移動する必要があります。まず、x座標のみを調べます。画像の上に薄い金属板を置いたところを想像してみてください。次に、各ソース点で、プレートのzオフセットが、そのソース点がターゲット位置に着地するためにx方向に移動する必要がある距離(正または負)になるように、プレートを曲げます。プレートは曲げに抵抗するため、滑らかなままです。ソース点以外の座標のオフセットは、プレートの位置から読み取ることができます。y座標についても同様の手順を繰り返すことができます。
これにより、任意の(x、y)座標をターゲット位置にマッピングする薄板スプラインモデルが得られます。
樽型歪みの修正#
この例では、薄板スプライン変換を使用して樽型歪み[3]を修正する方法を示します。樽型歪みは、画像の中心からの距離とともに画像の倍率が減少するという特徴的な魚眼効果を生み出します。
最初に、チェッカーボード画像に魚眼レンズの歪みを適用し、その後、逆の修正変換を適用することにより、データセットの例を生成します。
https://en.wikipedia.org/wiki/Distortion_(optics)#Radial_distortion
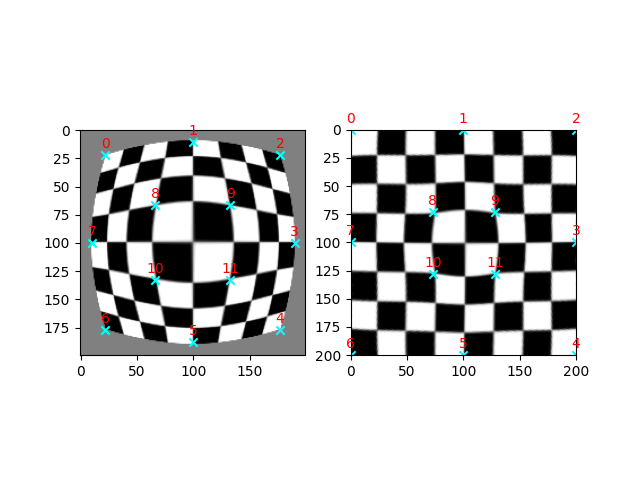
import matplotlib.pyplot as plt
import numpy as np
import skimage as ski
def radial_distortion(xy, k1=0.9, k2=0.5):
"""Distort coordinates `xy` symmetrically around their own center."""
xy_c = xy.max(axis=0) / 2
xy = (xy - xy_c) / xy_c
radius = np.linalg.norm(xy, axis=1)
distortion_model = (1 + k1 * radius + k2 * radius**2) * k2
xy *= distortion_model.reshape(-1, 1)
xy = xy * xy_c + xy_c
return xy
image = ski.data.checkerboard()
image = ski.transform.warp(image, radial_distortion, cval=0.5)
# Pick a few `src` points by hand, and move the corresponding `dst` points to their
# expected positions.
# fmt: off
src = np.array([[22, 22], [100, 10], [177, 22], [190, 100], [177, 177], [100, 188],
[22, 177], [ 10, 100], [ 66, 66], [133, 66], [ 66, 133], [133, 133]])
dst = np.array([[ 0, 0], [100, 0], [200, 0], [200, 100], [200, 200], [100, 200],
[ 0, 200], [ 0, 100], [ 73, 73], [128, 73], [ 73, 128], [128, 128]])
# fmt: on
# Estimate the TPS transformation from these points and then warp the image.
# We switch `src` and `dst` here because `skimage.transform.warp` requires the
# inverse transformation!
tps = ski.transform.ThinPlateSplineTransform()
tps.estimate(dst, src)
warped = ski.transform.warp(image, tps)
# Plot the results
fig, axs = plt.subplots(1, 2)
axs[0].imshow(image, cmap='gray')
axs[0].scatter(src[:, 0], src[:, 1], marker='x', color='cyan')
axs[1].imshow(warped, cmap='gray', extent=(0, 200, 200, 0))
axs[1].scatter(dst[:, 0], dst[:, 1], marker='x', color='cyan')
point_labels = [str(i) for i in range(len(src))]
for i, label in enumerate(point_labels):
axs[0].annotate(
label,
(src[:, 0][i], src[:, 1][i]),
textcoords="offset points",
xytext=(0, 5),
ha='center',
color='red',
)
axs[1].annotate(
label,
(dst[:, 0][i], dst[:, 1][i]),
textcoords="offset points",
xytext=(0, 5),
ha='center',
color='red',
)
plt.show()
スクリプトの総実行時間:(0分0.309秒)